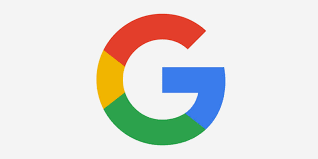
0
+
Google Reviews
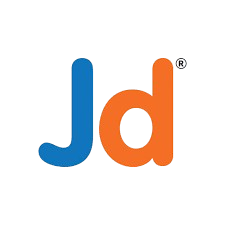
0
+
Empower your data-driven decisions with our Advanced Big Data Science Training in Kochi. Dive deep into cutting-edge techniques and tools for analyzing vast datasets. Gain hands-on experience in machine learning, predictive modeling, and data visualization. Learn from industry experts who provide practical insights and real-world applications. Collaborate with peers in dynamic workshops and projects to deepen your understanding. Prepare to tackle complex challenges and extract valuable insights from big data environments. Join us and embark on a transformative journey to become a proficient big data scientist in the thriving landscape of data analytics.
IBM Tivoli Storage Manager is a centralized, policy-based, enterprise class, data backup and recovery package. The software enables the user to insert objects not only via backup, but also through space management and archive tools. This application programming can help gigantic endeavor in various ways. The system has a critical impact in enormous endeavors in protecting every one of the information and help in recovering them when required. This decreases the danger of loss of data. The running with are couple of positive conditions offered by TSM server and Client.
Introduction To Data Science
Python Essentials (Core)
Data Manipulation – Cleansing – Munging Using Python Modules
Data Analysis – Visualization Using Python
Basic Statistics & Implementation Of Stats Methods In Python
Python: Machine Learning -Predictive Modeling – Basics
Machine Learning Algorithms & Applications – Implementation In Python
Project – Consolidate Learnings
Introduction To Big Data
Hadoop(Big Data) Eco-System
Hadoop Cluster-Architecture-Configuration Files
Hadoop-HDFS & MapReduce (YARN)
Data Integration Using Sqoop & Flume
Data Analysis Using Pig
Data Analysis Using Hive
Data Analysis Using Impala
Introduction To Other Ecosystem Tools
Spark: Introduction
Spark: Spark In Practice
Spark: Spark Meets Hive
Spark Streaming
Spark GraphX
Introduction To Machine Learning Using Spark
Project
+91 8882400500
I had undergone oracle DBA course under Chetan sir's Guidance an it was a very good learning experience overall since they not only provide us with theoretical knowledge but also conduct lot of practical sessions which are really fruitful and also the way of teaching is very fine clear and crisp which is easier to understand, overall I had a great time for around 2 months, they really train you well.also make it a point to clear all your doubts and provide you with clear and in-depth concepts hence hope to join sometime again
I have completed Oracle DBA 11g from Radical technology pune. Excellent trainer (chetna gupta). The trainer kept the energy level up and kept us interested throughout. Very practical, hands on experience. Gave us real-time examples, excellent tips and hints. It was a great experience with Radical technologies.
Linux learning with Anand sir is truly different experience... I don't have any idea about Linux and system but Anand sir taught with scratch...He has a great knowledge and the best trainer...he can solve all your queries related to Linux in very simple way and giving nice examples... 100 to Anand Sir.
I had a wonderful experience in Radical technologies where i did training in Hadoop development under the guidance of Shanit Sir. He started from the very basic and covered and shared everything he knew in this field. He was brilliant and had a lot of experience in this field. We did hands on for every topic we covered, and that's the most important thing because honestly theoretical knowledge cannot land you a job.
I have recently completed Linux course under Anand Sir and can assuredly say that it is definitely the best Linux course in Pune. Since most of the Linux courses from other sources are strictly focused on clearing the certification, they will not provide an insight into real-world server administration, but that is not the case with Anand Sir's course. Anand Sir being an experienced IT infrastructure professional has an excellent understanding of how a data center works and all these information is seamlessly integrated into his classes.
1. Basic user account management (creating, modifying, and deleting users).
2. Password resets and account unlocks.
3. Basic file system navigation and management (creating, deleting, and modifying files and directories).
4. Basic troubleshooting of network connectivity issues.
5. Basic software installation and package management (installing and updating software packages).
6. Viewing system logs and checking for errors or warnings.
7. Running basic system health checks (CPU, memory, disk space).
8. Restarting services or daemons.
9. Monitoring system performance using basic tools (top, df, free).
10. Running basic commands to gather system information (uname, hostname, ifconfig).
1. Intermediate user account management (setting permissions, managing groups).
2. Configuring network interfaces and troubleshooting network connectivity issues.
3. Managing file system permissions and access control lists (ACLs).
4. Performing backups and restores of files and directories.
5. Installing and configuring system monitoring tools (Nagios, Zabbix).
6. Analyzing system logs for troubleshooting purposes.
7. Configuring and managing software repositories.
8. Configuring and managing system services (systemd, init.d).
9. Performing system updates and patch management.
10. Monitoring and managing system resources (CPU, memory, disk I/O).
1. Advanced user account management (LDAP integration, single sign-on).
2. Configuring and managing network services (DNS, DHCP, LDAP).
3. Configuring and managing storage solutions (RAID, LVM, NFS).
4. Implementing and managing security policies (firewall rules, SELinux).
5. Implementing and managing system backups and disaster recovery plans.
6. Configuring and managing virtualization platforms (KVM, VMware).
7. Performance tuning and optimization of system resources.
8. Implementing and managing high availability solutions (clustering, load balancing).
9. Automating system administration tasks using scripting (Bash, Python).
10. Managing system configurations using configuration management tools (Ansible, Puppet).
1. Learning basic shell scripting for automation tasks. 2. Understanding file system permissions and ownership. 3. Learning basic networking concepts (IP addressing, routing). 4. Learning how to use package management tools effectively. 5. Familiarizing with common Linux commands and utilities. 6. Understanding basic system architecture and components. 7. Learning basic troubleshooting techniques and methodologies. 8. Familiarizing with basic security principles and best practices. 9. Learning how to interpret system logs and diagnostic output. 10. Understanding the role and importance of system backups and restores.
1. Advanced scripting and automation techniques (error handling, loops).
2. Understanding advanced networking concepts (VLANs, subnetting).
3. Familiarizing with advanced storage technologies (SAN, NAS).
4. Learning advanced security concepts and techniques (encryption, PKI).
5. Understanding advanced system performance tuning techniques.
6. Learning advanced troubleshooting methodologies (root cause analysis).
7. Implementing and managing virtualization and cloud technologies.
8. Configuring and managing advanced network services (VPN, IDS/IPS).
9. Implementing and managing containerization technologies (Docker, Kubernetes).
10. Understanding enterprise-level IT governance and compliance requirements.
1. Designing and implementing complex IT infrastructure solutions. 2. Architecting and implementing highly available and scalable systems. 3. Developing and implementing disaster recovery and business continuity plans. 4. Conducting security audits and vulnerability assessments. 5. Implementing and managing advanced monitoring and alerting systems. 6. Developing custom automation solutions tailored to specific business needs. 7. Providing leadership and mentorship to junior team members. 8. Collaborating with other IT teams on cross-functional projects. 9. Evaluating new technologies and making recommendations for adoption. 10. Participating in industry conferences, workshops, and training programs.
Abu Dhabi
Abu Dhabi
Abu Dhabi
Abu Dhabi
Abu Dhabi
Abu Dhabi
Abu Dhabi
Abu Dhabi
Abu Dhabi
Abu Dhabi
Abu Dhabi
Abu Dhabi
Abu Dhabi
Abu Dhabi
Abu Dhabi
Abu Dhabi
Abu Dhabi
Abu Dhabi
Abu Dhabi
Abu Dhabi
Abu Dhabi
Abu Dhabi
Abu Dhabi
Abu Dhabi
Abu Dhabi
Abu Dhabi
Abu Dhabi
Abu Dhabi
Abu Dhabi
Abu Dhabi
Abu Dhabi
Abu Dhabi
Abu Dhabi
Abu Dhabi
Abu Dhabi
Abu Dhabi
Abu Dhabi
Abu Dhabi
Abu Dhabi
Abu Dhabi
(Our Team will call you to discuss the Fees)
(Our Team will call you to discuss the Fees)